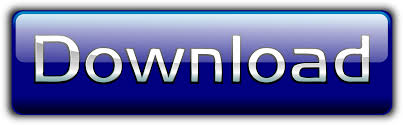
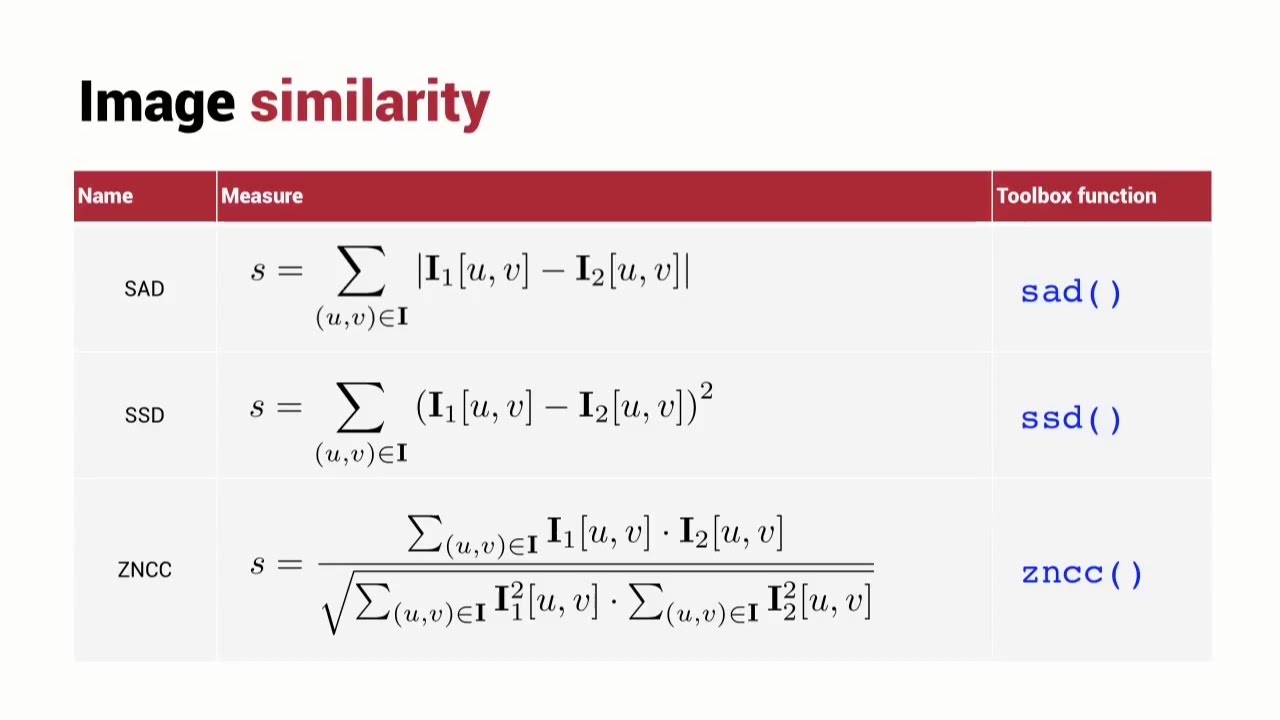
Finally we will identify the positions that yield the best similarity measures as the probable template occurrences. We will perform the actual search in a rather straightforward way – we will position the template over the image at every possible location, and each time we will compute some numeric measure of similarity between the template and the image segment it currently overlaps with. We are provided with a template image representing the reference object we are looking for and the input image to be inspected. Imagine that we are going to inspect an image of a plug and our goal is to find its pins. After that we will explain how this method is enhanced and extended in advanced Grayscale-based Matching and Edge-based Matching routines. We will start with a demonstration of a naive Template Matching method, which is insufficient for real-life applications, but illustrates the core concept from which the actual Template Matching algorithms stem from. Depending on the specific problem at hand, we may (or may not) want to identify the rotated or scaled occurrences. Template Matching techniques are expected to address the following need: provided a reference image of an object (the template image) and an image to be inspected (the input image) we want to identify all input image locations at which the object from the template image is present. Their applicability is limited mostly by the available computational power, as identification of big and complex templates can be time-consuming. Template Matching techniques are flexible and relatively straightforward to use, which makes them one of the most popular methods of object localization. Advanced template matching algorithms allow to find occurrences of the template regardless of their orientation and local brightness.
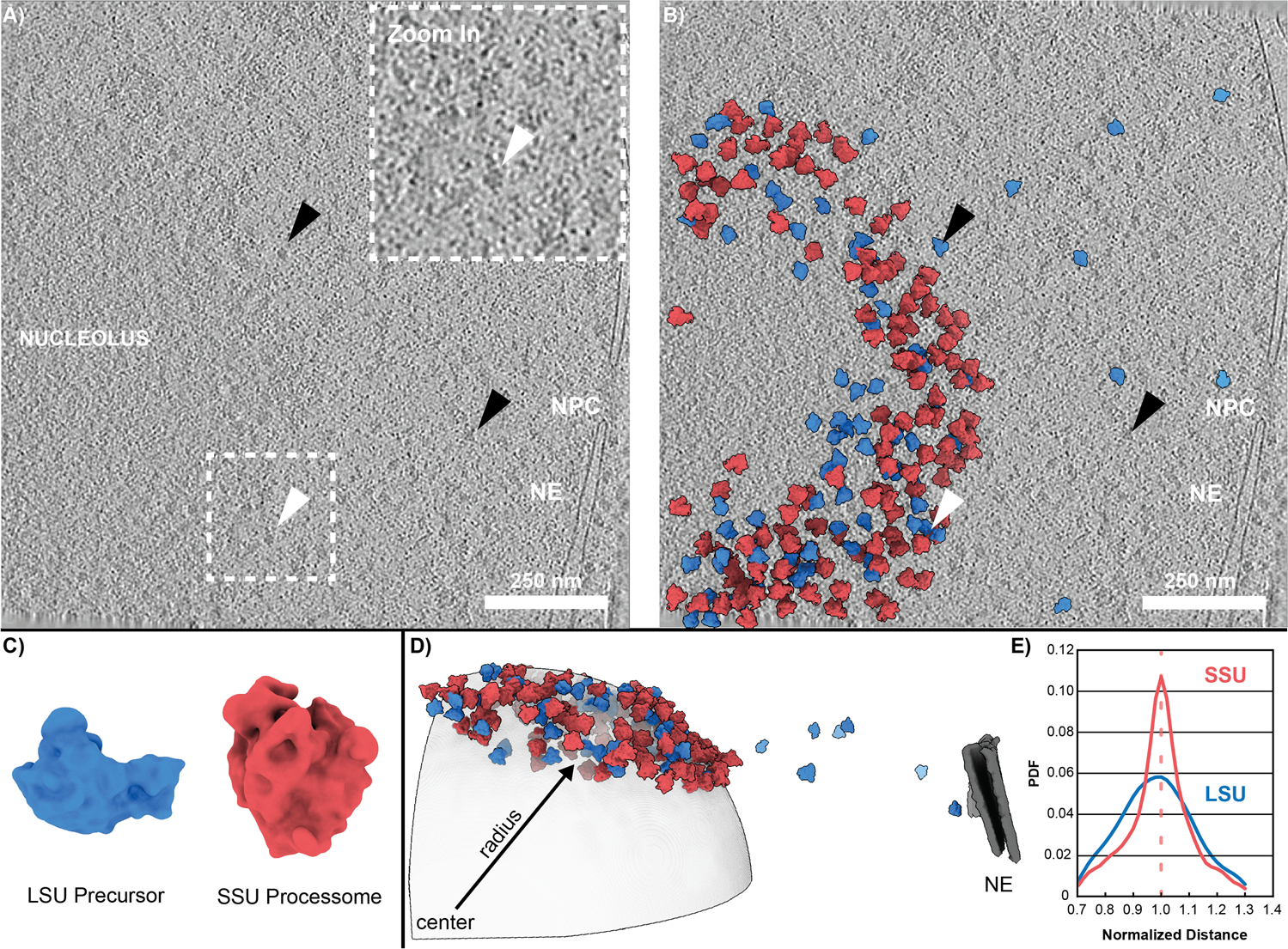
Template Matching is a high-level machine vision technique that identifies the parts on an image that match a predefined template. Template Matching Template Matching Introduction
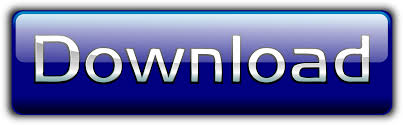